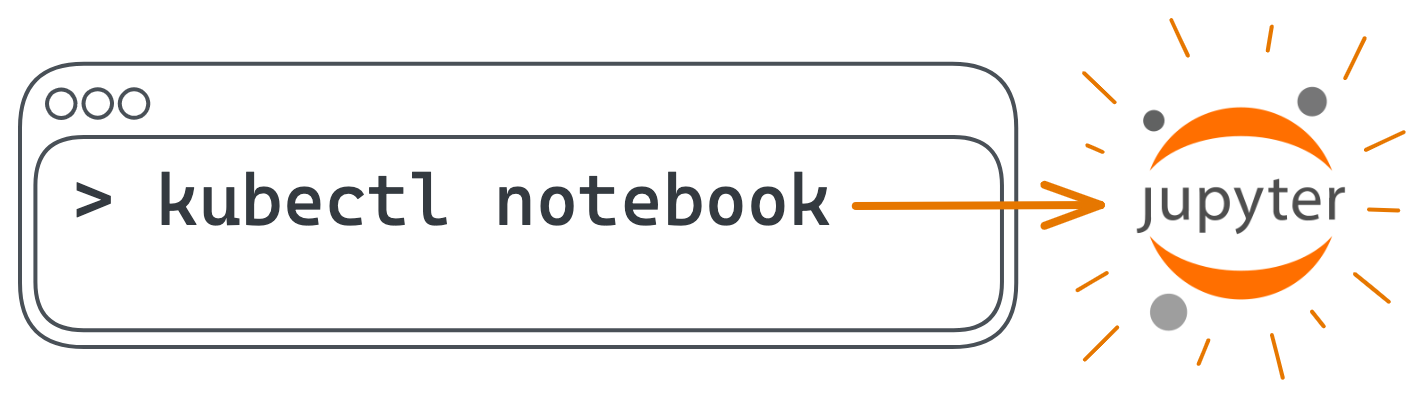
Substratus has added the kubectl notebook
command!
"Wouldn't it be nice to have a single command that containerized your local directory and served it as a Jupyter Notebook running on a machine with a bunch of GPUs attached?"
The conversation went something like that while we daydreamed about our preferred workflow. At that point in time we were hopping back-n-forth between Google Colab and our containers while developing a LLM training job.
"Annnddd it should automatically sync file-changes back to your local directory so that you can commit your changes to git and kick off a long-running ML training job - containerized with the exact same python version and packages!"
So we built it!
kubectl notebook -d .
And now it has become an integral part of our workflow as we build out the Substratus ML platform.
Check out the 50 second screenshare:
Design Goals
- One command should build, launch, and sync the Notebook.
- Users should only need a Kubeconfig - no other credentials.
- Admins should not need to setup networking, TLS, etc.
Implementation
We tackled our design goals using the following techniques:
- Implemented as a single Go binary, executed as a kubectl plugin.
- Signed URLs allow for users to upload their local directory to a bucket without requiring cloud credentials (Similar to how popular consumer clouds function).
- Kubernetes port-forwarding allows for serving remote notebooks without requiring admins to deal with networking / TLS concerns. It also leans on existing Kubernetes RBAC for access control.
Some interesting details:
- Builds are executed remotely for two reasons:
- Users don't need to install docker.
- It avoids pushing massive container images from one's local machine (pip installs often inflate the final docker image to be much larger than the build context itself).
- The client requests an upload URL by specifying the MD5 hash it wishes to upload - allowing for server-side signature verification.
- Builds are skipped entirely if the MD5 hash of the build context already exists in the bucket.
The system underneath the notebook
command:
More to come!
Lazy-loading large models from disk... Incremental dataset loading... Stay tuned to learn more about how Notebooks on Substratus can speed up your ML workflows.
Don't forget to star and follow the repo!
https://github.com/substratusai/substratus
Star